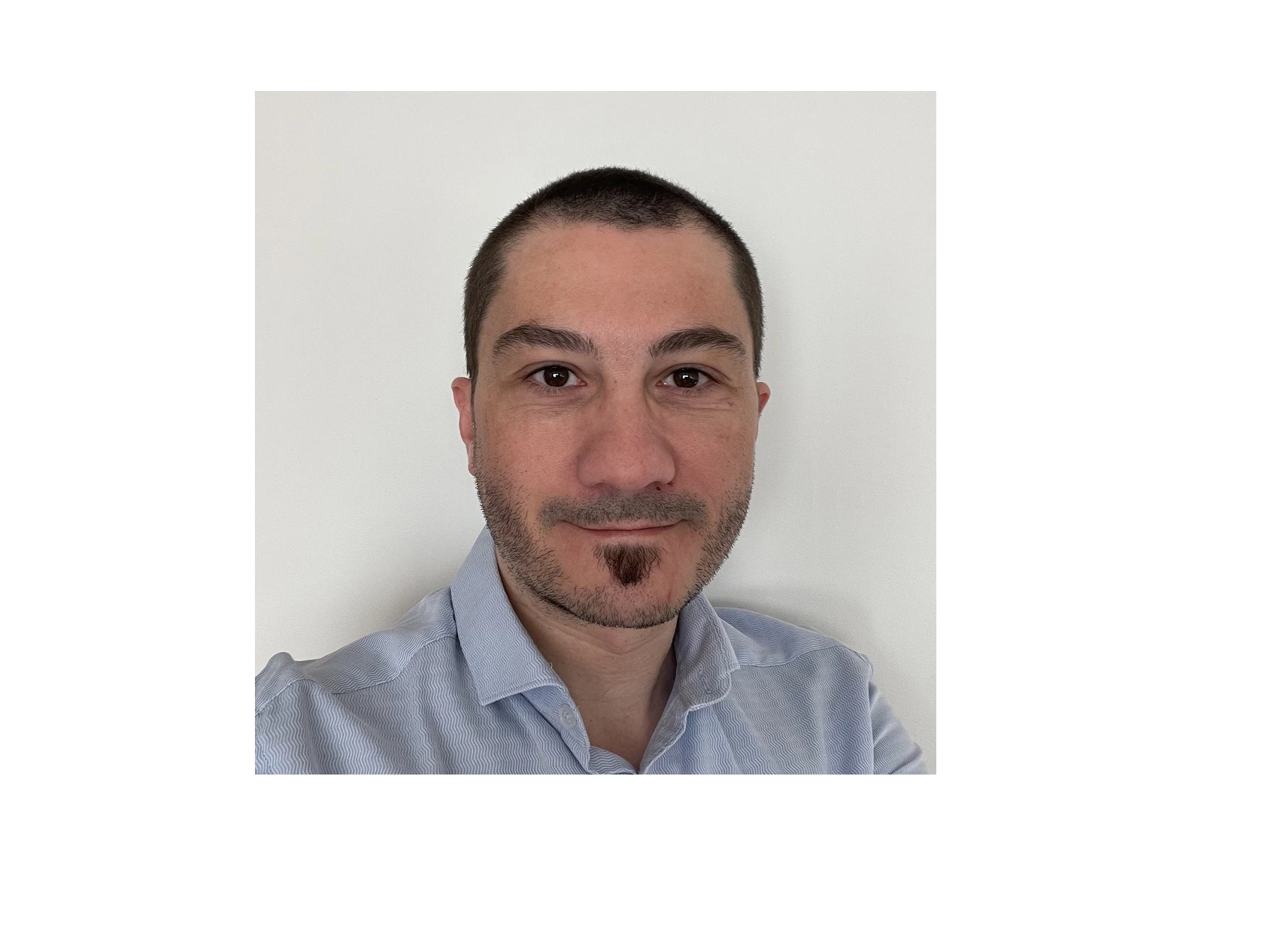
Partner Article
Why quality data is behind the best business decisions
Patrick Peinoit, Principal Product Manager, Talend
Data quality is at the very heart of organizations’ concerns, but why do we constantly associate it with words like “challenge”, “problem” or “barriers”? This is probably because of the increase in awareness around the criticality of a data quality strategy. If we are to succeed in data transformation, to become truly data-driven, data quality cannot be a “nice to have” any more. On the contrary, it must become the foundation for any data initiatives.
While data quality is becoming a core discipline of data, we still haven’t fully mastered how to understand it.
Collaboration One of the key points of achieving data quality is that it must become a collaborative process. In particular, it has become increasingly important to include the business lines in the process. Businesses can first, treat data quality like a team sport where it can be managed and processed. Second, combine the business users’ understanding of the objectives and purposes of data use with the required control and governance provided by IT teams. In an ideal world, this collaborative process would achieve a level of integration and communication similar to the model of Google Suite or Office 365, with gateways everywhere, providing ease of use for business users.
For larger organizations, scale becomes critical. To standardize data quality and roll it out on a large scale, self-service solutions have been developed to improve collaboration. With data preparation or data stewardship solutions, users have control over the data they need and can apply the necessary rules and ensure data availability, while IT teams manage data governance and access needs. However, whenever new processes are introduced, some data tools are still more difficult to understand than others. Often at the first obstacle, business users will hurriedly revert to Excel and PowerPoint. And with a lack of understanding of data and limited initiatives to increase levels of “data literacy,” organizations inevitably find themselves with the kind of silos that many organizations are familiar with.
Culture and Contextualization In the data sector, there are two parallel universes that struggle to connect: the teams who process the data and those who use it. The former understand processing, but do not understand what they are processing; they do not “speak data” in the same way the business user does.
What needs to be done is to introduce a data culture, and finally start to consider data as information that must be established. Implementing a data quality project is really more than just a matter of tools. The actual mindset of the company needs to change, and team members need to share the same understanding of the information. Data quality is contextual. This is where a cohesive culture around data becomes important. Team members will measure its data according to certain criteria and certain dimensions. But these criteria are rarely enough in themselves.
Let’s take the example of completeness of information - the data either exists or it doesn’t exist. But is it really a problem if the data doesn’t exist?
We are all familiar with the example of customer information in a database with “opt-in” and “opt-out” fields. If the customer is “opt-in”, we can find their phone number, for example, but if the customer is “opt-out”, we should not be able to find any personal information about them. The information in fact must not be available, and it is actually its very absence that makes the data “valid” with regard to the legislation on data privacy. The definition of information completeness is therefore only possible with some context regarding the information.
Certain technologies and tools play an important role in contextualizing data. This is the case with metadata that allows users to find the data, know if the data exists, and understand the data. We can use metadata in data cataloging and data inventory tools, and the more metadata we have, the better our understanding of the data will be, and as a result, our understanding of the information generated. Rule repository, data preparation and data stewardship technologies also play a central role in contextualization, because they allow us to apply rules and transform raw data into contextualized information for the business user.
If we’re finally going to treat our data as a genuine corporate asset, then we need to start by understanding it.
This was posted in Bdaily's Members' News section by Talend .
Enjoy the read? Get Bdaily delivered.
Sign up to receive our popular morning National email for free.